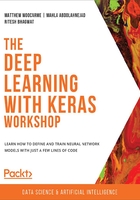
Summary
In this chapter, we covered the various types of linear algebra components and operations that pertain to machine learning. These components include scalars, vectors, matrices, and tensors. The operations that were applied to these tensors included addition, transposition, and multiplication—all of which are fundamental for understanding the underlying mathematics of ANNs.
We also learned some of the basics of the Keras package, including the mathematics that occurs at each node. We replicated the model from the previous chapter, in which we built a logistic regression model to predict the same target from the online shopping purchasing intention dataset. However, in this chapter, we used the Keras library to create the model using an ANN instead of the scikit-learn logistic regression model. We achieved a similar level of accuracy using ANNs.
The upcoming chapters of this book will use the same concepts we learned about in this chapter; however, we will continue building ANNs with the Keras package. We will extend our ANNs to more than a single layer by creating models that have multiple hidden layers. By adding multiple hidden layers to our ANNs, we will put the "deep" into "deep learning". We will also tackle the issues of underfitting and overfitting since they are related to training models with ANNs.