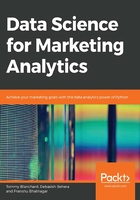
Customer Segmentation Methods
Customer segmentation is the act of separating (segmenting) your target customers into different groups based on demographic or behavioral data so that marketing strategies can be tailored more specifically to each group. Being able to accurately segment a customer population is becoming increasingly important in today's digital world, where products and advertisements are created to target more and more specific subsets of the population. It is also an important part of allocating marketing resources properly because, by targeting specific customer groups, you can achieve higher return on investment for your marketing initiatives.
Every marketing group does some amount of customer segmentation. However, the methods they use to do this might not always be clear. These may be based on intuitions and hunches about certain demographic groups, or they might be the output of some marketing software, where the methods used are actually obscure. There are advantages and disadvantages of every possible method, and understanding them allows you to make use of the right tool for the job. In the following sections, we will discuss some of the most commonly used approaches for customer segmentation and also discuss their pros and cons.
Traditional Segmentation Methods
There are different methods for performing customer segmentation. Probably the most common general method is for a marketing analyst to sit down with the data they have about customers, whether it's demographic or behavioral, and to try to come up with rough groupings based on intuitions and arbitrary thresholds. An example of this would be deciding to segment customers into different income tiers, based on $10,000 increments.
These kinds of methods have the advantage of being simple and easy to understand. However, this becomes much more complex when you add additional variables. Importantly, as you increase the number of groups in the number of variables, it becomes hard to choose thresholds in a way such that you don't end up with groups with very few customers in them. For example, how many individuals would be there in the group "18- to 25-year-olds making $100,000+"?
This becomes more important when looking at the behavioral data of customers. Creating groups based on intuition can result in underlying patterns in the data being overlooked. For example, there may be segments of the population that respond well to very specific kinds of marketing offers. If the analyst performing the segmentation doesn't happen to know about this specific group and the types of ads they respond to, they may miss out on capturing them as a unique group. For instance, a marketing analyst who separates customers into those who respond to offers for expensive products and those who respond to offers for inexpensive products could miss a group of customers only interested in electronics, regardless of whether they are expensive or inexpensive.
Unsupervised Learning (Clustering) for Customer Segmentation
Another method for performing customer segmentation is using unsupervised learning. Using unsupervised learning is often a very powerful technique as it tends to pick up on patterns in data that might otherwise be missed. It's perfect for customer segmentation because it finds data points that are most like each other and groups them together, which is exactly what good customer segmentation techniques should do.
Clustering is a type of unsupervised machine learning that looks for groups or "clusters" in data without knowing them ahead of time. The following are some of the advantages and disadvantages of using clustering for customer segmentation.
Here are the advantages of clustering:
- Can find customer groups that are unexpected or unknown to the analyst
- Flexible and can be used for a wide range of data
- Reduces the need for deep expertise about connections between the demographics of customers and behaviors
- Quick to perform; scalable to very large datasets
Here are the disadvantages of clustering:
- Customer groups created may not be easily interpretable.
- If data is not based on consumer behavior (such as products or services purchased), it may not be clear how to use the clusters that are found.
As you can see, one downside of clustering is that it may find groups that don't seem to make a lot of sense on the surface. Often this can be fixed by using a better suited clustering algorithm. Determining how to evaluate and fine-tune clustering algorithms will be the topic of our next chapter.