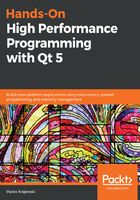
Profiling to Find Bottlenecks
As we already described in Chapter 1, Understanding Performant Programs, performance bottlenecks are not distributed evenly in code. Applying the Pareto principle to performance optimization, we could say that 80% of a program's execution time is spent on 20% of the code (and, as we've seen, Donald Knuth believed this to be an even lower figure). Regardless of the exact proportions, the basic insight is the same—code optimization efforts are most effectively spent on that critical x percent of the code that is responsible for most of the program's execution time.
We saw in the previous chapter that today's hardware platforms have grown to be so complicated that the ancient adage about programmers being notoriously bad at estimating bottlenecks in their own code has now been reinforced more than ever—not only do we either understand our code, but, we don't understand the hardware on which it runs anymore either! In Chapter 1, Understanding Performant Programs, we introduced the notion of premature optimization as the process of blindly optimizing pieces of software without obtaining any performance data, and thus, insights relying on gut feeling only. So, this chapter will be about tooling, which enables us to measure and obtain that data. The topics discussed will include the following:
- Types of profilers: Some preliminary knowledge about our tools
- Platform and tools: Because we don't want to stick to theory alone
- Profiling CPU usage: Let's put our tooling to use
- Investigating memory usage: Putting more tooling to work
- Going further-advanced tools: Because there's always another tool
Let's start with what performance data is, what we are able to measure, and how can we do that.