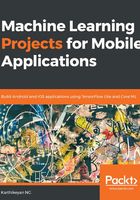
Age, gender, and emotion prediction
This chapter is going to cover a complete iOS application using Core ML models to detect age, gender, and emotion from a photo taken using an iPhone camera or from a photo in a user's phone gallery.
Core ML enables developers to install and run pre-trained models on a device, and this has its own advantages. Since Core ML lives in the local device, it is not necessary to call a cloud service in order to get the prediction results. This improves the communication latency and also saves data bandwidth. The other crucial benefit of Core ML is privacy. You don't need to send your data to a third party in order to get the results picked for you. The main downside of having an offline model is that the model cannot be updated, and so it cannot be improved with newer inputs. Furthermore, a few models might increase memory footprints, since storage is limited on a mobile device.
This project is built on a MacBook Pro machine with Xcode version 9.3 on macOS High Sierra. Age and gender prediction became a common application on social media platforms. While there are multiple algorithms for predicting and classifying age and gender, these are still being improved in terms of performance. In this chapter, the classification is done based on deep CNNs.