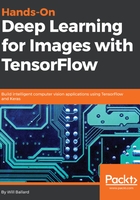
What this book covers
Chapter 1, Machine Learning Toolkit, looks into installing Docker, setting up a machine learning Docker file, sharing data back with your host computer, and running a REST service to provide the environment.
Chapter 2, Image Data, teaches MNIST digits, how to acquire them, how tensors are really just multidimensional arrays, and how we can encode image data and categorical or classification data as a tensor. Then, we have a quick review and a cookbook approach to consider dimensions and tensors, in order to get data prepared for machine learning.
Chapter 3, Classical Neural Network, covers an awful lot of material! We see the structure of the classical, or dense, neural network. We learn about activation, nonlinearity, and softmax. We then set up testing and training data and learn how to construct the network with Dropout and Flatten. We also learn all about solvers, or how machine actually learns. We then explore hyperparameters, and finally, we fine-tune our model by means of grid search.
Chapter 4, A Convolutional Neural Network, teaches you convolutions, which are a loosely connected way of moving over an image to extract features. Then we learn about pooling, which summarizes the most important features. We will build a convolutional neural network using these techniques and we combine many layers of convolution and pooling in order to generate a deep neural network.
Chapter 5, An Image Classification Server, uses a Swagger API definition to create a REST API model, which then declaratively generates the Python framework in order for us to serve that API. Then, we create a Docker container that captures not only our running code (that is, our service) but also our pre-trained machine learning model. This then forms a package so that we are able to deploy and use our container. Finally, we use this container to serve and make predictions.