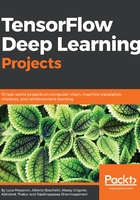
The TensorFlow object detection API
As a way of boosting the capabilities of the research community, Google research scientists and software engineers often develop state-of-the-art models and make them available to the public instead of keeping them proprietary. As described in the Google research blog post, https://research.googleblog.com/2017/06/supercharge-your-computer-vision-models.html , on October 2016, Google's in-house object detection system placed first in the COCO detection challenge, which is focused on finding objects in images (estimating the chance that an object is in this position) and their bounding boxes (you can read the technical details of their solution at https://arxiv.org/abs/1611.10012).
The Google solution has not only contributed to quite a few papers and been put to work in some Google products (Nest Cam - https://nest.com/cameras/nest-aware/, Image Search - https://www.blog.google/products/search/now-image-search-can-jump-start-your-search-style/, and Street View - https://research.googleblog.com/2017/05/updating-google-maps-with-deep-learning.html), but has also been released to the larger public as an open source framework built on top of TensorFlow.
The framework offers some useful functions and these five pre-trained different models (constituting the so-called pre-trained Model Zoo):
- Single Shot Multibox Detector (SSD) with MobileNets
- SSD with Inception V2
- Region-Based Fully Convolutional Networks (R-FCN) with Resnet 101
- Faster R-CNN with Resnet 101
- Faster R-CNN with Inception Resnet v2
The models are in growing order of precision in detection and slower speed of execution of the detection process. MobileNets, Inception and Resnet refer to different types of CNN network architectures (MobileNets, as the name suggests, it is the architecture optimized for mobile phones, smaller in size and faster in execution). We have discussed CNN architecture in the previous chapter, so you can refer there for more insight on such architectures. If you need a refresher, this blog post by Joice Xu can help you revise the topic in an easy way: https://towardsdatascience.com/an-intuitive-guide-to-deep-network-architectures-65fdc477db41.
Single Shot Multibox Detector (SSD), Region-Based Fully convolutional networks (R-FCN) and Faster Region-based convolutional neural networks (Faster R-CNN) are instead the different models to detect multiple objects in images. In the next paragraph, we are going to explain something about how they effectively work.
Depending on your application, you can decide on the most suitable model for you (you have to experiment a bit), or aggregate results from multiple models in order to get better results (as done by the researchers at Google in order to win the COCO competition).