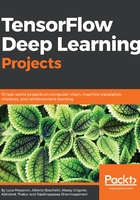
Summary
This project has helped you to start immediately classifying objects in images with confidence without much hassle. It helps you to see what a ConvNet could do for your problem, focusing more on the wrap up (possibly a larger application) you have in mind,and annotating many images for training more ConvNets with fresh images of a selected class.
During the project, you have learned quite a few useful technicalities you can reuse in many projects dealing with images. First of all, you now know how to process different kinds of visual inputs from images, videos, and webcam captures. You also know how to load a frozen model and put it to work, and also how to use a class to access a TensorFlow model.
On the other hand, clearly, the project has some limitations that you may encounter sooner or later, and that may spark the idea to try to integrate your code and make it shine even more. First of all, the models we have discussed will soon be surpassed by newer and more efficient ones (you can check here for newly available models: https://github.com/tensorflow/models/blob/master/object_detection/g3doc/detection_model_zoo.md), and you will need to incorporate new ones or create your own architecture (https://github.com/tensorflow/models/blob/master/object_detection/g3doc/defining_your_own_model.md). Then you may need to combine the model to reach the accuracy you need in your project (the paper Speed/accuracy trade-offs for modern convolutional object detectors reveals how researchers at Google have done it). Finally, you may need to tune a ConvNet to recognize a new class (you can read how to do that here, but beware, it is a long process and a project by itself: https://github.com/tensorflow/models/blob/master/object_detection/g3doc/using_your_own_dataset.md).
In the next chapter, we will look at state-of-the-art object detection in images, devising a project that will lead you to produce complete discursive captions describing submitted images, not just simple labels and bounding boxes.