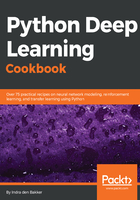
上QQ阅读APP看书,第一时间看更新
Using PyTorch’s dynamic computation graphs for RNNs
PyTorch is the Python deep learning framework and it's getting a lot of traction lately. PyTorch is the Python implementation of Torch, which uses Lua. It is backed by Facebook and is fast thanks to GPU-accelerated tensor computations. A huge benefit of using PyTorch over other frameworks is that graphs are created on the fly and are not static. This means networks are dynamic and you can adjust your network without having to start over again. As a result, the graph that is created on the fly can be different for each example. PyTorch supports multiple GPUs and you can manually set which computation needs to be performed on which device (CPU or GPU).