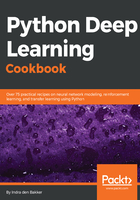
Implementing a single-layer neural network
Now we can move on to neural networks. We will start by implementing the simplest form of a neural network: a single-layer neural network. The difference from a perceptron is that the computations are done by multiple units (neurons), hence a network. As you may expect, adding more units will increase the number of problems that can be solved. The units perform their computations separately and are stacked in a layer; we call this layer the hidden layer. Therefore, we call the units stacked in this layer the hidden units. For now, we will only consider a single hidden layer. The output layer performs as a perceptron. This time, as input we have the hidden units in the hidden layer instead of the input variables:

In our implementation of the perceptron, we've used a unit step function to determine the class. In the next recipe, we will use a non-linear activation function sigmoid for the hidden units and the output function. By replacing the step function with a non-linear activation function, the network will be able to uncover non-linear patterns as well. More on this later in the Activation functions section. In the backward pass, we use the derivative of the sigmoid to update the weights.
In the following recipe, we will classify two non-linearly separable classes with NumPy.