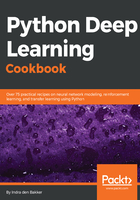
Experiment with hidden layers and hidden units
The most commonly used layers in general neural networks are fully-connected layers. In fully-connected layers, the units in two successive layers are all pairwise connected. However, the units within a layer don't share any connections. As stated before, the connections between the layers are also called trainable parameters. The weights of these connections are trained by the network. The more connections, the more parameters and the more complex patterns can be modeled. Most state-of-the-art models have 100+ million parameters. However, a deep neural network with many layers and units takes more time to train. Also, with extremely deep models the time to infer predictions takes significantly longer (which can be problematic in a real-time environment). In the following chapters, we will introduce other popular layer types that are specific to their network types.
Picking the correct number of hidden layers and hidden units can be important. When using too few nodes, the model won't be able to pick up all the signals, resulting in a low accuracy and poor predictive performance (underfitting). Using too many nodes, the model will tend to overfit on the training data (see regularization for techniques to prevent overfitting) and won't be able to generalize well. Therefore, we always have to look at the performance on the validation data to find the right balance. In the next recipe, we will show an example of overfitting and output the number of trainable parameters.