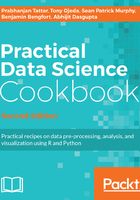
上QQ阅读APP看书,第一时间看更新
How to do it...
The following steps will lead you through the initial exploration of our dataset, where we compute some of its basic parameters:
- First, let's find out how many observations (rows) are in our data:
nrow(vehicles)
## 34287
- Next, let's find out how many variables (columns) are in our data:
ncol(vehicles)
## 74
- Now, let's get a sense of which columns of data are present in the data frame using the names function:
> names(vehicles)
The preceding command will give you the following output:

Luckily, a lot of these column or variable names are pretty descriptive and give us an idea of what they might contain. Remember, a more detailed description of the variables is available at http://www.fueleconomy.gov/feg/ws/index.shtml#vehicle.
- Let's find out how many unique years of data are included in this dataset by computing a vector of the unique values in the year column, and then computing the length of that vector:
length(unique(vehicles[, "year"]))
## 31
- Now we determine the first and last years present in the dataset using the min and max functions:
first_year <- min(vehicles[, "year"])
## 1984
last_year <- max(vehicles[, "year"])
## 2014
Note that we could have used the tail command, which would have displayed the last few rows of the data frame instead of the first few rows.
- Also, since we might use the year variable a lot, let's make sure that we have each year covered. The list of years from 1984 to 2014 should contain 31 unique values. To test this, use the following command:
> length(unique(vehicles$year))
[1] 31
- Next, let's find out what types of fuel are used as the automobiles' primary fuel types:
table(vehicles$fuelType1)
## Diesel Electricity Midgrade Gasoline Natural Gas
## 1025 56 41 57
## Premium Gasoline Regular Gasoline
## 8521 24587
From this, we can see that most cars in the dataset use regular gasoline, and the second most common fuel type is premium gasoline.
- Let's explore the types of transmissions used by these automobiles. We first need to take care of all missing data by setting it to NA:
vehicles$trany[vehicles$trany == ""] <- NA
- Now, the trany column is text, and we only care about whether the car's transmission is automatic or manual. Thus, we use the substr function to extract the first four characters of each trany column value and determine whether it is equal to Auto. If so, we set a new variable, trany2, equal to Auto; otherwise, the value is set to Manual:
vehicles$trany2 <- ifelse(substr(vehicles$trany, 1, 4) == "Auto",
"Auto", "Manual")
- Finally, we convert the new variable to a factor and then use the table function to see the distribution of values:
vehicles$trany <- as.factor(vehicles$trany)
table(vehicles$trany2)
## Auto Manual
## 22451 11825
We can see that there are roughly twice as many automobile models with automatic transmission as there are models with manual transmission.