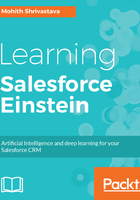
Data
In the DASE architecture, data is prepared by two components sequentially--Data Source and Data Preparator.
Data Source takes data from the data store and prepares an RDD [Rating] for the ALS algorithm. Consider the following diagram:

The Data Preparator sample looks as follows:
package org.template.recommendation;
import org.apache.predictionio.controller.java.PJavaPreparator;
import org.apache.spark.SparkContext;
public class Preparator extends PJavaPreparator<TrainingData,
PreparedData> {
@Override
public PreparedData prepare(SparkContext sc,
TrainingData trainingData) {
return new PreparedData(trainingData);
}
}
The PreparedData class returns TrainingData.
The sample Java class for DataSource consists of the method named readTraining(), which can read the event's and create Training Data based on those events.
The sample Java code looks as follows:
public class DataSource extends PJavaDataSource<TrainingData,
EmptyParams, Query, Set<String>> {
private final DataSourceParams dsp;
public DataSource(DataSourceParams dsp) {
this.dsp = dsp;
}
@Override
public TrainingData readTraining(SparkContext sc) {
JavaRDD<UserItemEvent> viewEventsRDD = PJavaEventStore.find(
dsp.getAppName(),
OptionHelper.<String>none(),
OptionHelper.<DateTime>none(),
OptionHelper.<DateTime>none(),
OptionHelper.some("user"),
OptionHelper.<String>none(),
OptionHelper.some(Collections.singletonList("view")),
OptionHelper.<Option<String>>none(),
OptionHelper.<Option<String>>none(),
sc)
.map(new Function<Event, UserItemEvent>() {
@Override
public UserItemEvent call(Event event) throws Exception {
return new UserItemEvent(event.entityId(),
event.targetEntityId().get(),
event.eventTime().getMillis(), UserItemEventType.VIEW);
}
});
}
--
return new TrainingData(usersRDD, itemsRDD, viewEventsRDD,
buyEventsRDD);
}
The key things to note from the preceding code are as follows:
- data is loaded from the event store using the engine object, PJavaEventStore
- Refer to the PredictionIO Scala documents for more information around the PJavaEventStore methods at (http://predictionio.incubator.apache.org/api/current/#org.apache.predictionio.data.store.java.PJavaEventStore$)
- Refer to the Spark Documentation to deep dive into the Function method (https://spark.apache.org/docs/latest/api/java/index.html?org/apache/spark/api/java/function/package-summary.html)
Scala version of the preceding code is shown next.
The Preparator class sample is as follows:
package MyRecommedationScala
import org.apache.predictionio.controller.PPreparator
import org.apache.spark.SparkContext
import org.apache.spark.SparkContext._
import org.apache.spark.rdd.RDD
class Preparator extends PPreparator[TrainingData, PreparedData] {
def prepare(sc: SparkContext, trainingData: TrainingData):
PreparedData = {
new PreparedData(
users = trainingData.users,
items = trainingData.items,
viewEvents = trainingData.viewEvents,
buyEvents = trainingData.buyEvents)
}
}
class PreparedData(
val users: RDD[(String, User)],
val items: RDD[(String, Item)],
val viewEvents: RDD[ViewEvent],
val buyEvents: RDD[BuyEvent]
) extends Serializable
The DataSource class reads the events and creates TrainingData based on the following events:
class DataSource(val dsp: DataSourceParams)
extends PDataSource[TrainingData,
EmptyEvaluationInfo, Query, EmptyActualResult] {
@transient lazy val logger = Logger[this.type]
override
def readTraining(sc: SparkContext): TrainingData = {
val eventsRDD: RDD[Event] = PEventStore.find(
appName = dsp.appName,
entityType = Some("user"),
eventNames = Some(List("view", "buy")),
// targetEntityType is optional field of an event.
targetEntityType = Some(Some("item")))(sc).cache()
val viewEventsRDD: RDD[ViewEvent] = eventsRDD
.filter { event => event.event == "view" }
.map { ... }
...
new TrainingData(...)
}
}