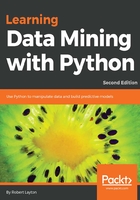
Evaluating the association rules
In a broad sense, we can evaluate the association rules using the same concept as for classification. We use a test set of data that was not used for training, and evaluate our discovered rules based on their performance in this test set.
To do this, we will compute the test set confidence, that is, the confidence of each rule on the testing set. We won't apply a formal evaluation metric in this case; we simply examine the rules and look for good examples.
Formal evaluation could include a classification accuracy by determining the accuracy of predicting whether a user rates a given movie as favorable. In this case, as described below, we will informally look at the rules to find those that are more reliable:
- First, we extract the test dataset, which is all of the records that we didn't use in the training set. We used the first 200 users (by ID value) for the training set, and we will use all of the rest for the testing dataset. As with the training set, we will also get the favorable reviews for each of the users in this dataset as well. Let's look at the code:
test_dataset = all_ratings[~all_ratings['UserID'].isin(range(200))]
test_favorable = test_dataset[test_dataset["Favorable"]]
test_favorable_by_users = dict((k, frozenset(v.values)) for k, v in
test_favorable.groupby("UserID")["MovieID"])
- We then count the correct instances where the premise leads to the conclusion, in the same way that we did before. The only change here is the use of the test data instead of the training data. Let's look at the code:
correct_counts = defaultdict(int)
incorrect_counts = defaultdict(int)
for user, reviews in test_favorable_by_users.items():
for candidate_rule in candidate_rules:
premise, conclusion = candidate_rule
if premise.issubset(reviews):
if conclusion in reviews:
correct_counts[candidate_rule] += 1
else:
incorrect_counts[candidate_rule] += 1
- Next, we compute the confidence of each rule from the correct counts and sort them. Let's look at the code:
test_confidence = {candidate_rule:
(correct_counts[candidate_rule] / float(correct_counts[candidate_rule] + incorrect_counts[candidate_rule]))
for candidate_rule in rule_confidence}
sorted_test_confidence = sorted(test_confidence.items(), key=itemgetter(1), reverse=True)
- Finally, we print out the best association rules with the titles instead of the movie IDs:
for index in range(10):
print("Rule #{0}".format(index + 1))
premise, conclusion = sorted_confidence[index][0]
premise_names = ", ".join(get_movie_name(idx) for idx in premise)
conclusion_name = get_movie_name(conclusion)
print("Rule: If a person recommends {0} they will also recommend {1}".format(premise_names, conclusion_name))
print(" - Train Confidence: {0:.3f}".format(rule_confidence.get((premise, conclusion), -1)))
print(" - Test Confidence: {0:.3f}".format(test_confidence.get((premise, conclusion), -1)))
print("")
We can now see which rules are most applicable in new unseen data:
Rule #1
Rule: If a person recommends Shawshank Redemption, The (1994), Silence of the Lambs, The (1991), Pulp Fiction (1994), Star Wars (1977), Twelve Monkeys (1995) they will also recommend Raiders of the Lost Ark (1981)
- Train Confidence: 1.000
- Test Confidence: 0.909
Rule #2
Rule: If a person recommends Silence of the Lambs, The (1991), Fargo (1996), Empire Strikes Back, The (1980), Fugitive, The (1993), Star Wars (1977), Pulp Fiction (1994) they will also recommend Twelve Monkeys (1995)
- Train Confidence: 1.000
- Test Confidence: 0.609
Rule #3
Rule: If a person recommends Silence of the Lambs, The (1991), Empire Strikes Back, The (1980), Return of the Jedi (1983), Raiders of the Lost Ark (1981), Twelve Monkeys (1995) they will also recommend Star Wars (1977)
- Train Confidence: 1.000
- Test Confidence: 0.946
Rule #4
Rule: If a person recommends Shawshank Redemption, The (1994), Silence of the Lambs, The (1991), Fargo (1996), Twelve Monkeys (1995), Empire Strikes Back, The (1980), Star Wars (1977) they will also recommend Raiders of the Lost Ark (1981)
- Train Confidence: 1.000
- Test Confidence: 0.971
Rule #5
Rule: If a person recommends Shawshank Redemption, The (1994), Toy Story (1995), Twelve Monkeys (1995), Empire Strikes Back, The (1980), Fugitive, The (1993), Star Wars (1977) they will also recommend Return of the Jedi (1983)
- Train Confidence: 1.000
- Test Confidence: 0.900
The second rule, for instance, has a perfect confidence in the training data, but it is only accurate in 60 percent of cases for the test data. Many of the other rules in the top 10 have high confidences in test data, making them good rules for making recommendations.
You may also notice that these movies tend to be very popular and good films. This gives us a baseline algorithm that we could compare against, i.e. instead of trying to do personalized recommendations, just recommend the most liked movies overall. Have a shot at implementing this algorithm - does the Apriori algorithm outperform it and by how much? Another baseline could be to simply recommend movies at random from the same genre.
If you are looking through the rest of the rules, some will have a test confidence of -1. Confidence values are always between 0 and 1. This value indicates that the particular rule wasn't found in the test dataset at all.