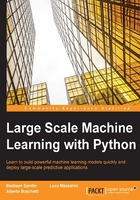
Chapter 2. Scalable Learning in Scikit-learn
Loading a dataset into memory, preparing a data matrix, training a machine learning algorithm, and testing its generalization capabilities using out-of-sample observations are often not such a big deal given the quite powerful and yet affordable computers of this day and age. However, more and more frequently, the scale of the data to be elaborated is so huge that loading it into the core memory of your computer is not possible and, even if manageable, the result is intractable both in terms of data management and machine learning.
Alternative viable strategies beyond the core memory processing are possible: splitting the data into samples, using parallelism, and finally learning in small batches or by single instances. The present chapter will focus on the out-of-the-box solution that the Scikit-learn package offers: the streaming of mini batches of instances (our observations) from data storage and the incremental learning based on them. Such a solution is called out-of-core learning.
To treat the data by working on manageable chunks and learning incrementally is a great idea. However, when you try to implement it, it can also prove challenging because of the limitations in the available learning algorithms and streaming data in a flow will require you to think differently in terms of data management and feature extraction. Beyond presenting the Scikit-learn functionalities for out-of-core learning, we will also strive to present you with Python solutions for apparently daunting problems you can face when forced to observe only small portions of your data at a time.
In this chapter, we will cover the following topics:
- The way out-of-core learning is implemented in Scikit-learn
- Effectively managing streams of data using the hashing trick
- The nuts and bolts of stochastic learning
- Implementing data science with online learning
- Unsupervised transformations of streams of data