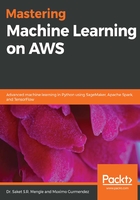
Naive Bayes – pros and cons
In this section, we present the advantages and disadvantages in selecting the Naive Bayes algorithm for classification problems.
These are the pros:
Training time: The Naive Bayes algorithm only requires one pass on the entire dataset to calculate the posterior probabilities for each value of the feature in the dataset. So, when we are dealing with large datasets or low-budget hardware, the Naive Bayes algorithm is a feasible choice for most data scientists.
Prediction time: Since all the probabilities are pre-computed in the Naive Bayes algorithm, the prediction time of this algorithm is very efficient.
Transparency: Since the predictions of Naive Bayes algorithms are based on the posterior probability of each conditional feature, it is easy to understand which features are influencing the predictions. This helps users to understand the predictions.
These are the cons:
Prediction accuracy: The prediction accuracy of the Naive Bayes algorithm is lower than other algorithms we will discuss in the book. Algorithm prediction accuracy is dataset dependent. A lot of research has proved that algorithms such as random forest, support vector machines (SVMs), and deep neural networks (DNNs) outperform the Naive Bayes algorithm in terms of classification accuracy.
Assumption of independence: Since we assume that the features are independent of each other, this algorithm may lose information for features that are dependent on each other. Other advanced algorithms do use this dependence information when calculating predictions.