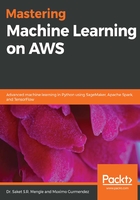
Summary
In this chapter, we explained why ML is a crucial tool in a data scientist's repository. We discussed what a structured ML dataset looks like and how to identify the types of features in the dataset.
We took a deep dive into the Naive Bayes classification algorithm, and studied how Bayes' theorem is used in the Naive Bayes algorithm. We learned that, using Bayes' theorem, we can predict the probability of an event occurring based on the values of each feature, and select the event that has the highest probability.
We also presented an example of a Twitter dataset. We hope that you learned how to think about a text classification problem, and how to build a Naive Bayes classification model to predict the source of a tweet. We also presented how the algorithm can be implemented in SageMaker, and how it can also be implemented using Apache Spark. This code base should help you tackle any text classification problems in the future. As the implementation is presented using SageMaker services and Spark, it can scale to datasets that can be gigabytes or terabytes in size.
We will look at how to deploy the ML models on actual production clusters in later chapters.